12/12 o3 Saturates the ARC-AGI Benchmark!
OpenAI impresses with the capabilities of its newest reasoning models
All intuition about AI capabilities will need to get updated for o3.
Good morning!
OpenAI just revealed two new, very impressive reasoning models, o3 and o3-mini. These models set records on some of the most challenging STEM benchmarks, such as EpochAI Frontier Math, AIME 2024, SWE-Bench-Verified, and Codeforces. Surprisingly, even the popular ARC-AGI benchmark got saturated!
EpochAI Frontier Math consists of questions that even Field medalists (the most prestigious award in mathematics) would need hours or days to solve. The previous state-of-the-art accuracy was 2%. Now, o3 achieves 25.2%.
AIME is the second exam in the series to challenge students to choose the team representing the United States at the International Mathematics Olympiad (IMO). On the AIME 2024 test, o1 achieved 56.6% accuracy. o3 now scores an impressive 96.7%.
The most surprising result is o3's performance on the ARC-AGI benchmark, introduced by François Chollet in 2019. He co-created it to evaluate models' overall intelligence. It comprises tasks that are easy for humans to solve but that AI models of the past could simply not. Read François's blog post for more details. These newly trained models' results show how OpenAI’s test time compute paradigm matters and is here to stay.
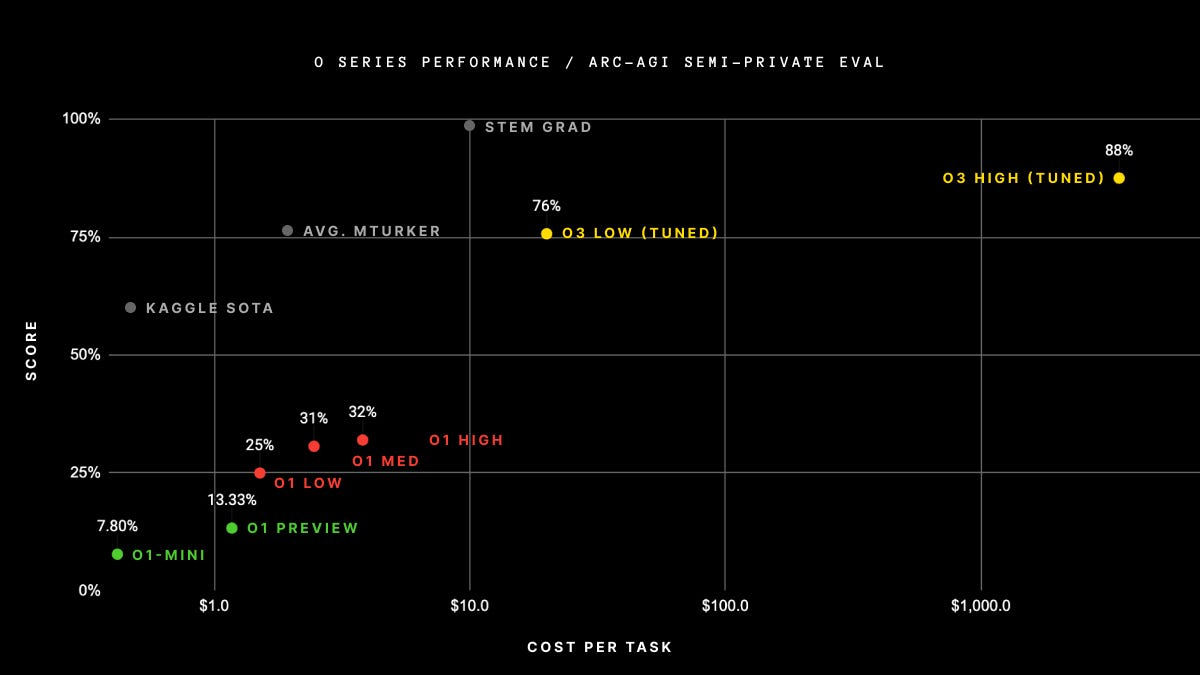
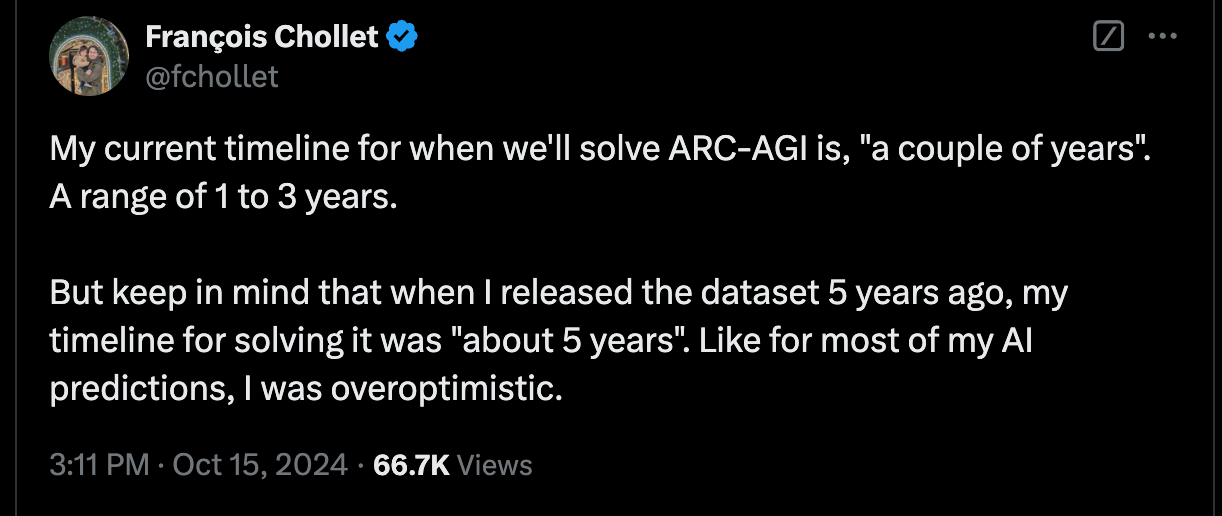
Two days ago, OpenAI announced a 60% reduction in token usage for the reasoning component. This means the models are now cheaper and faster to use.
A graph shown during the demo indicated that o1-mini’s response time is not far off from GPT-4o. We expect this trend of better reasoning with reduced latency to continue improving over the coming years. We also know that o3-mini will outperform o1 on coding tasks at a massive cost reduction!
In terms of token efficiency, François Chollet writes:
Of course, such generality comes at a steep cost, and wouldn't quite be economical yet: you could pay a human to solve ARC-AGI tasks for roughly $5 per task (we know, we did that), while consuming mere cents in energy. Meanwhile o3 requires $17-20 per task in the low-compute mode (and 172x compute for the high mode, the blog shows o3 exceeding $1K per query). But cost-performance will likely improve quite dramatically over the next few months and years, so you should plan for these capabilities to become competitive with human work within a fairly short timeline.
It's astonishing to reflect on how far we’ve come in just one year. Back then, projects like MetaGPT on GitHub tackled the limitations of earlier models like GPT-4 by creating multi-agent frameworks to automate software development. These frameworks were novel, enabling the generation of complex projects like a full 2048 game, an Angry Birds clone, and many others.
Now, with models like o1 and o3, these complex systems are no longer necessary to build these “simple” projects. You can achieve similar or even better results with a single prompt without needing the overhead of multi-agent systems. The rate of improvement is so fast. It’s hard to imagine where we’ll be in a few more years if this pace continues.
That’s it for the 12 days of Christmas of OpenAI! We didn’t quite get a bingo, but we made some great predictions and came close on others — maybe we were just a bit too hopeful. The past 12 days have been a mix of exciting new AI models, enhanced capabilities, and OpenAI products challenging the big tech companies
.